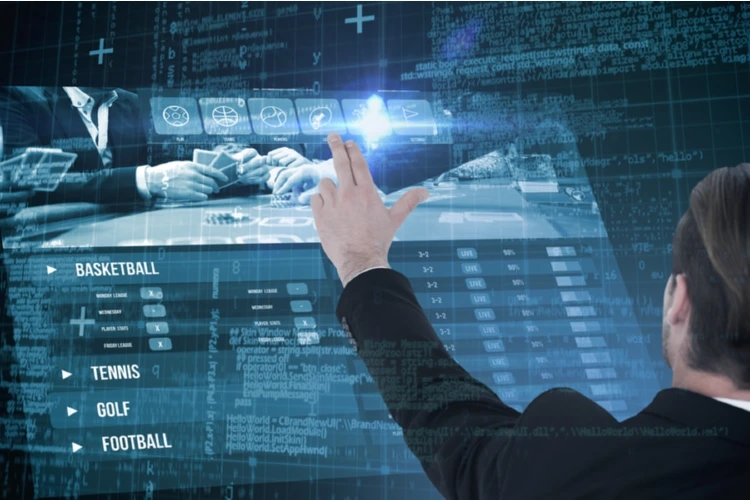
In the ever-evolving landscape of football analysis and betting strategies, a relatively new concept has emerged that is revolutionizing the way we understand the beautiful game: expected goals, commonly referred to as xG.
In this comprehensive guide, we will delve deep into what xG is, how it works, and most importantly, how you can leverage it to enhance your betting experience.
Understanding xG
Expected Goals (xG) is a statistical metric that quantifies the quality of scoring chances in a football match. It assigns a numerical value to each shot based on various factors such as shot location, shot type, angle, and proximity to the goal, among others. The fundamental idea behind xG is to provide a more accurate assessment of a team’s attacking performance and goal-scoring potential than traditional metrics like goals scored.
xG is significant in football analytics because it offers a more nuanced understanding of a team’s offensive efficiency. While the number of goals scored in a match is essential, it doesn’t always reflect the true balance of play or the quality of scoring opportunities created. For example, a team may score multiple goals in a match but have a low xG value if those goals came from low-quality chances or fortunate circumstances. Conversely, a team may have a high xG value despite scoring few goals if they consistently create high-quality scoring opportunities.
By quantifying the likelihood of a goal being scored from a particular shot, xG allows analysts, coaches, and fans to evaluate a team’s attacking performance objectively. It helps identify areas for improvement, assess the effectiveness of different playing styles, and compare teams’ offensive outputs accurately. Moreover, xG can be used to predict future match outcomes and assess player and team performance over a more extended period.
The origins of xG: How did this metric come about, and why is it gaining traction?
The concept of expected goals originated from the field of sports analytics, where statisticians and researchers sought to develop more sophisticated methods for evaluating football matches beyond traditional statistics. The idea can be traced back to the early 2000s, but it gained prominence in the following decade with the advent of advanced data collection technologies and the availability of large-scale football databases.
The rise of xG can also be attributed to the growing demand for data-driven insights in football, both among clubs and fans. As the sport became increasingly commercialized and competitive, teams sought ways to gain a competitive edge by leveraging data and analytics to optimize player recruitment, tactical strategies, and performance analysis. Likewise, fans and media outlets began embracing advanced statistics as a means of enhancing their understanding and enjoyment of the game.
Today, xG has become a mainstream concept in football analytics, with its usage extending beyond academic research and into practical applications within clubs, media coverage, and betting markets. Its growing popularity can be attributed to its intuitive appeal and demonstrated effectiveness in providing valuable insights into the intricacies of football performance.
Components of xG: Breaking down the factors that contribute to expected goals
Several factors contribute to the calculation of expected goals for a particular shot:
1. Shot Location: The position on the pitch from which the shot was taken significantly influences its likelihood of resulting in a goal. Shots taken from central areas closer to the goal typically have a higher xG value than those taken from wider angles or longer distances.
2. Shot Type: Different shot types, such as headers, volleys, or shots from open play versus set-pieces, are assigned varying xG values based on historical data on their success rates.
3. Angle and Proximity to Goal: The angle at which the shot is taken and its distance from the goal are crucial determinants of its xG value. Shots taken from acute angles or further away from the goal have lower xG values compared to those taken from more advantageous positions.
4. Defensive Pressure: The presence of defenders and the goalkeeper in the vicinity of the shooter can affect the difficulty of the shot and, consequently, its xG value. Shots taken under minimal defensive pressure are more likely to result in goals than those taken under tight marking or with multiple defenders blocking the shooting lane.
By considering these factors in conjunction with historical data on shot outcomes, xG models can generate accurate estimates of the likelihood of a shot resulting in a goal. This enables analysts and coaches to assess the quality of scoring opportunities objectively and make data-informed decisions to improve team performance.
How xG is Calculated?
Expected Goals (xG) is calculated using various methodologies and models developed by analysts to quantify the likelihood of a shot resulting in a goal. These models take into account a range of factors, including shot location, shot type, assists, defensive pressure, and other variables, to generate accurate estimates of a shot’s expected outcome. Moreover, advanced machine learning algorithms are increasingly being employed to refine xG models and enhance their predictive accuracy.
Methodologies behind xG calculations: Exploring the various models used by analysts
There are several methodologies and models used by analysts to calculate xG, each with its own approach and intricacies. Some of the commonly employed methods include:
1. Expected Goals (xG) Models: These models assign a numerical value to each shot based on its likelihood of resulting in a goal. They typically consider factors such as shot location, shot type, angle, and distance from the goal, among others. Examples of popular xG models include Opta’s xG model, StatsBomb’s xG model, and Expected Threat (xT) models.
2. Machine Learning Algorithms: Machine learning techniques, such as regression analysis, neural networks, and decision trees, are used to develop xG models that can learn and adapt from large datasets of historical shot data. These algorithms analyze thousands of shots to identify patterns and relationships between various input variables and shot outcomes, enabling them to generate more accurate xG predictions.
3. Hybrid Models: Some analysts combine multiple xG models or incorporate additional features, such as player and team attributes, match context, and defensive strategies, into their calculations to improve the robustness and predictive power of their xG models. These hybrid models aim to capture a broader range of factors that influence a shot’s likelihood of resulting in a goal.
Overall, the methodologies behind xG calculations vary depending on the specific model and approach used by analysts. While there is no one-size-fits-all solution, the common goal is to develop accurate and reliable xG metrics that provide valuable insights into the quality of scoring chances in football matches.
Factors considered in xG calculations: Shot location, shot type, assists, and other variables:
In calculating xG, analysts consider a range of factors that influence a shot’s likelihood of resulting in a goal. These factors include:
1. Shot Location: The position on the pitch from which the shot is taken is a critical determinant of its xG value. Shots taken from central areas closer to the goal typically have higher xG values than those taken from wider angles or further away from the goal.
2. Shot Type: Different shot types, such as headers, volleys, or shots from open play versus set-pieces, are assigned varying xG values based on historical data on their success rates. For example, shots taken from inside the penalty area generally have higher xG values than shots taken from outside the box.
3. Assists: The involvement of an assisting player in creating a scoring opportunity can influence the xG value of a shot. Assisted shots, where the shooter receives a pass or cross from a teammate, may have higher xG values than unassisted shots due to the increased likelihood of a successful goal attempt.
4. Defensive Pressure: The presence of defenders and the goalkeeper in the vicinity of the shooter can affect the difficulty of the shot and, consequently, its xG value. Shots taken under minimal defensive pressure are more likely to result in goals than those taken under tight marking or with multiple defenders blocking the shooting lane.
Machine learning and xG: How advanced algorithms are used to refine xG model
Machine learning algorithms play a crucial role in refining xG models and enhancing their predictive accuracy. These algorithms analyze large datasets of historical shot data to identify patterns, trends, and relationships between various input variables and shot outcomes. By training on vast amounts of data, machine learning models can learn and adapt over time, improving their ability to predict a shot’s likelihood of resulting in a goal.
Some of the advanced machine learning techniques used in xG modeling include:
1. Regression Analysis: Linear and logistic regression models are commonly used to analyze the relationship between input variables (e.g., shot location, shot type) and shot outcomes (e.g., goal scored or not). These models estimate the probability of a shot resulting in a goal based on the observed data, allowing analysts to calculate xG values for individual shots.
2. Neural Networks: Deep learning algorithms, such as artificial neural networks, are capable of learning complex patterns and relationships in data by simulating the structure and function of the human brain. Neural networks can be trained on large datasets of shot data to develop highly accurate xG models that can capture nonlinear relationships and interactions between input variables.
3. Decision Trees: Decision tree algorithms partition the input space into hierarchical decision rules based on the values of input variables, allowing analysts to predict a shot’s outcome based on a series of binary decisions. Ensemble methods, such as random forests and gradient boosting, combine multiple decision trees to improve predictive performance and robustness.
Why xG Matters in Football?
While goals and assists are essential metrics in football, they have inherent limitations when it comes to evaluating player and team performance comprehensively. Goals, for example, only capture the end result of attacking sequences and do not account for the quality of scoring opportunities created. A team may score multiple goals in a match, but if those goals come from low-quality chances or lucky deflections, they may not accurately reflect the team’s attacking prowess.
Similarly, assists only consider the final pass leading directly to a goal and may overlook the contributions of other players in the build-up to the scoring opportunity. Players who consistently make key passes or create goal-scoring chances for their teammates may not receive recognition for their contributions if they do not register assists.
By contrast, expected goals (xG) provides a more nuanced and comprehensive assessment of attacking performance by quantifying the quality of scoring opportunities created. It considers factors such as shot location, shot type, defensive pressure, and other variables to estimate the likelihood of a shot resulting in a goal. This allows analysts, coaches, and fans to evaluate a team’s attacking efficiency objectively and identify areas for improvement beyond traditional metrics like goals and assists.
Implementing xG in Betting Strategies
xG and betting: the combination of football analytics and sports betting.
The integration of expected goals (xG) into betting strategies represents a significant evolution in the intersection of football analytics and sports wagering. While traditional betting approaches often rely on subjective factors or historical statistics like goals scored, xG offers a more objective and data-driven approach to assessing match outcomes. By quantifying the quality of scoring opportunities and evaluating team performance more comprehensively, xG provides valuable insights that can inform smarter betting decisions.
Bettors can leverage xG data to gain a deeper understanding of a team’s attacking and defensive capabilities, identify potential mismatches, and assess the likelihood of specific outcomes, such as the number of goals scored or the result of a match. This allows them to identify value bets—those with favorable odds relative to their perceived likelihood of occurring—and capitalize on inefficiencies in the betting markets.
Leveraging xG for smarter betting decisions: Identifying value bets and assessing match outcomes:
One of the key benefits of incorporating xG into betting strategies is its ability to identify value bets that offer favorable risk-reward opportunities. By comparing a team’s expected goals (xG) output to the betting odds offered by bookmakers, bettors can identify instances where there is a discrepancy between the perceived likelihood of a particular outcome and its actual probability based on xG data.
For example, if a team consistently generates high xG values but has relatively long odds to win a match, it may present an attractive betting opportunity, as the odds may not accurately reflect the team’s true goal-scoring potential. Conversely, if a team’s xG output suggests they are overperforming relative to their underlying performance level, betting against them may offer value despite their short odds.
Moreover, xG can help bettors assess the likely outcome of a match more accurately by providing insights into the quality of scoring opportunities created by each team. By considering factors such as shot location, shot type, and defensive pressure, bettors can evaluate the likelihood of specific outcomes, such as the number of goals scored or the result of a match, and make more informed betting decisions accordingly.
Finally, the advent of expected goals (xG) signifies a dramatic change in the field of sports betting and football analysis. By offering a more objective and complex evaluation of teams’ attacking performances, xG offers insightful information that can help bettors make better choices and increase their overall success when it comes to sports betting.