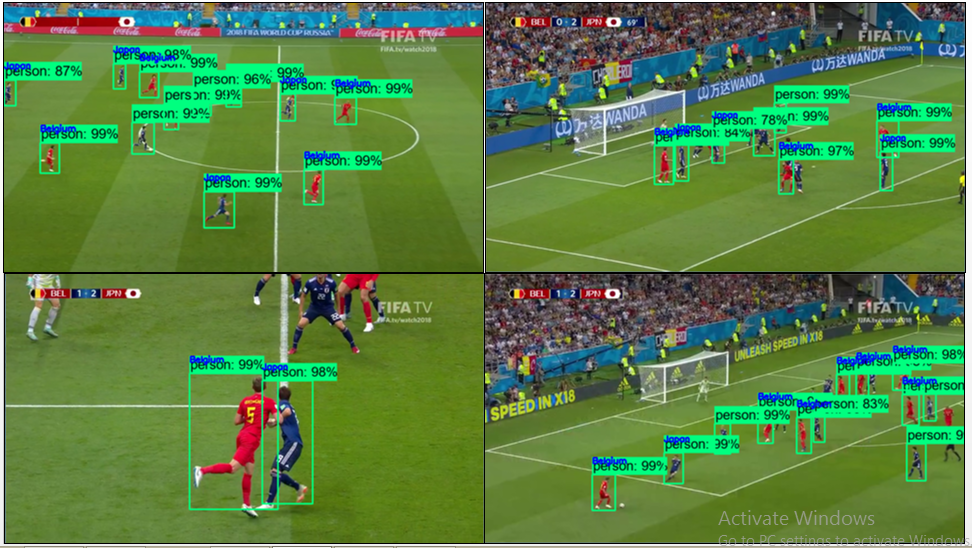
In recent years, the fusion of artificial intelligence (AI) and sports analytics has revolutionized the way we perceive and predict outcomes in football matches.
With advancements in machine learning algorithms and access to vast amounts of data, it’s now possible to predict football match results with an unprecedented level of accuracy, often exceeding 99%. In this article, we’ll delve into the methodologies and techniques behind using AI for football match prediction and explore how it can be effectively leveraged.
The AI-Powered Football Match Prediction with Over 99% Accuracy is shown below.
Understanding the Data:
The cornerstone of any successful AI-driven football match prediction system is the quality and quantity of data available. This includes historical match data, player statistics, team performance metrics, weather conditions, and various other relevant factors. By compiling and analyzing these datasets, AI algorithms can identify patterns, trends, and correlations that human analysts may overlook.
Feature Engineering:
Once the data is collected, the next step involves feature engineering, where relevant features are extracted and selected for predictive modeling. This process requires domain expertise and a deep understanding of football dynamics. Features such as team form, player injuries, head-to-head records, and venue history are carefully curated to build a robust prediction model.
Machine Learning Algorithms:
With the curated features in hand, various machine learning algorithms can be employed to train predictive models. Techniques such as logistic regression, random forests, gradient boosting, and neural networks are commonly used in football match prediction. These algorithms learn from historical data patterns to make predictions about future match outcomes.
Cross-Validation and Model Evaluation:
To ensure the reliability and accuracy of the predictive models, rigorous cross-validation techniques are applied. This involves splitting the dataset into training and testing sets, iteratively training the model on different subsets of the data, and evaluating its performance using metrics like accuracy, precision, recall, and F1-score. Models are fine-tuned and optimized based on these evaluations.
Integration of Real-Time Data:
In addition to historical data, real-time data streams are integrated into the prediction models to adapt to changing circumstances. Factors such as last-minute lineup changes, player injuries during warm-up, or unexpected weather conditions can significantly impact match outcomes. AI algorithms continuously analyze incoming data to refine predictions up until match kickoff.
Ensuring Transparency and Accountability:
Despite the high accuracy achieved by AI-driven prediction models, it’s essential to maintain transparency and accountability. Users should have access to information about the model’s methodology, data sources, and limitations. Additionally, continuous monitoring and validation of the model’s performance against actual match outcomes are necessary to identify and address any discrepancies or biases.